New Release
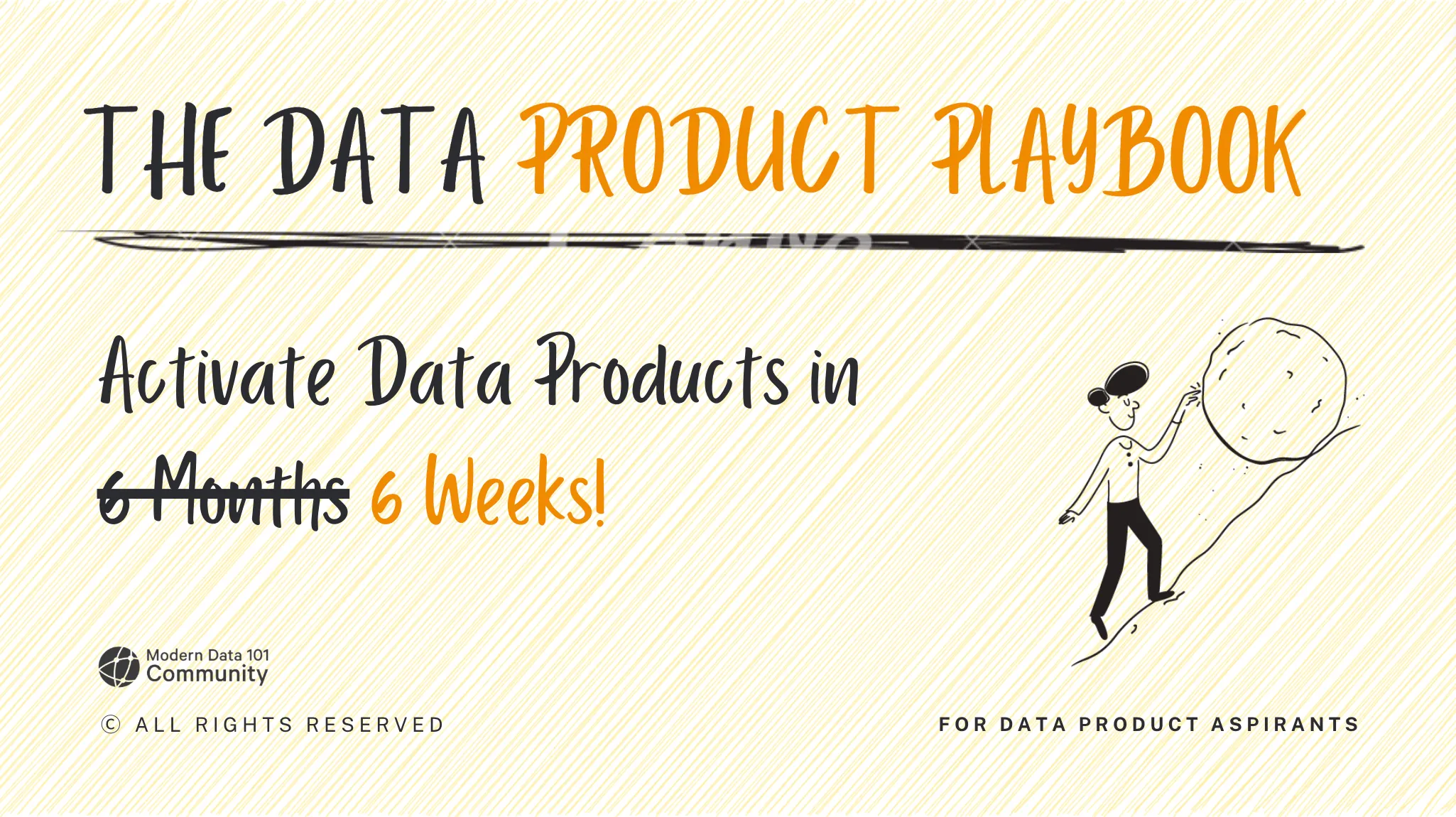
Learn More
Your submission has been received!
Thank you for submitting!
Thank you for submitting!
Download your PDF
Oops! Something went wrong while submitting the form.
Table of Contents
Get weekly insights on modern data delivered to your inbox, straight from our hand-picked curations!
the following is a revised edition.
Marketing Analytics is the backbone of growth in the marketing domain. This is the root of decisions, initiatives, and performance. Driving decisions backed by data creates an incredibly significant and lasting impact on marketing operations, ROI attributions, and Year-on-Year goals.
~ CMO of Upkeep.com (source: cmoalliance.com)
However, as with all data operations, marketing analytics also goes through deep-rooted challenges, which makes “data-driven” a distant goal rather than a reality. And realistically, a domain can only go so far without data-backed strategies.
Each time I was searching for a new tool to come into the place, it meant that every single tool needs to be connected one to another…We were investing so much money in all these tools, and we realized that some people were not actually using them. Something I've been saying all the time, a tool is going to show the return on investment at the moment people are going to use it.…And we actually ditched some tools down the way. Tools were great, in my opinion, but the response was not there, and we had to close them down.”
~ CMO of TRAPO Asia (source: cmoalliance.com)
We’ll not dive deep into the pains of data and analytics engineering within the scope of this piece. Later in the article, we’ve covered a few high-level pain points. Here are a few more references that might add more context to these. Feel free to check it out.
Let’s dive into the solution here. How to close the gap between data and business through internal monetisation of data and create more impact on marketing north stars such as High Conversions, High Revenue & ARR impact, High YoY Growth, Low Customer Acquisition Cost, and more.
But before jumping into the solution, let’s understand the marketing landscape first. Roughly, this is a bunch of typical marketing functions of mid- to large-sized orgs.
Note how these functions and the tools residing in the functions are all interrelated (addressed below under “integrated with”). Ideal scenario: leveraging this network of touchpoints through a consistent data stream. Existing scenario: Functions operating in silos and lack of tech-enabled cross-channel exchange.
Enables the delivery and management of ads across various digital channels like search engines, social media, and websites.
Tool Stack (examples): Google Ads, Meta Ads Manager, AdRoll, DV360
Integrated with: Social Media Marketing, Email Marketing, CRM, Content Marketing
Manages content and advertising across social platforms, helping engage audiences and build brand awareness.
Tool Stack (examples): Hootsuite, Buffer, Sprout Social, Facebook Ads
Integrated with: Advertising, CRM, Content Marketing
Sends targeted emails to prospects and customers, focusing on nurturing relationships and driving conversions.
Tool Stack (examples): Mailchimp, HubSpot, ActiveCampaign
Integrated with: CRM, Content Marketing, Experiential Marketing, ABM
Develops valuable, relevant content to attract and engage a target audience with the goal of driving profitable customer action.
Tool Stack (examples): WordPress, HubSpot, SEMrush
Integrated with: SEO Management, SMM, CRM, Email Marketing, Advertising
Optimizes content and websites for search engines to improve visibility and rankings.
Tool Stack (examples): Google Search Console, Ahrefs, Moz, SEMrush
Integrated with: Content Marketing
Targets specific accounts with personalised campaigns to drive engagement and conversions.
Tool Stack: Demandbase, HubSpot, Marketo
Integrated with: CRM, Experiential Marketing, Email Marketing
Creates immersive experiences that allow customers to engage with brands in memorable, tangible ways.
Tool Stack: Splash, Eventbrite, HubSpot, Qualtrics
Integrated with: Account-based Marketing, Email Marketing
Stores and manages customer data, facilitating communication between marketing and sales teams and driving lead conversions.
Examples: Salesforce, HubSpot, Zoho CRM
Integrated with: Content Marketing, ABM, Email Marketing, Sales
Now that we have a rough understanding of the landscape let’s consider the journey of a typical prospect from the unknown to the interested state. This is one of many possible pathways as a quick reference and touches various functions at the same time.
The unaware prospect comes across one of the campaigns by the brand. This could either be an email, social, or advertising campaign. These campaigns, again, can be owned by different functions. For example, an email campaign by the account-based marketing team or an advertising campaign by content marketing.
There are two possibilities: either the prospect chooses to engage with the campaign or ignores it. Let’s go with scenario A. This action leads to a diverse range of activities. At this stage, the prospect is only warmed up at best and casually browses resources that might be beneficial only on an awareness or self-development level. This indicates engagement with available content, social media, website pages, or similar.
Once the prospect’s trust develops further, they might sign up (willingly share contact) for exclusive or regular insights from the brand, say, through newsletters or exclusive ebooks, guides, or other gated resources. This may recur more than once until trust is solidified into consideration.
On entering “consideration”, the prospect is willing to engage with not just generic resources but is also willing to explore product-specific options they could imbibe into their lives. This means engaging with webinars/podcasts or product-specific content to understand if it’s worth a slice of time. If the answer is yes, they go in for a demo, and the brand has a lead 🏆
Here’s a much more detailed illustration of a prospect’s journey from being “only aware” to “an advocate of the brand”.
Here’s the Martech map for reference.
One might argue, “Aren’t marketing analytics tools enough to analyse and make data operational?”
Each chosen tool doesn’t just solve a part of the process but also spews out data as a by-product. While primary data assets are considered, such as website performance data on Google Analytics, all the by-products either aren’t integrated well enough to give a holistic prospect view, or they are entirely excluded from the data model.
Even if we choose to keep the by-products on the sidelines and just go with primary data assets, data integration is a persistent challenge that results in subsequent issues.
Marketing data resides in multiple platforms (social media, email, CRM, advertising tools), making it difficult to integrate. Lacking unified integration = incomplete or incorrect insights. This also implies challenges in cross-channel integration and is primarily caused by technology fragmentation, where different teams use different tools for campaign execution, tracking, and analysis.
The touchpoints a prospect goes through are so spread out across the marketing channels that from a distance, it might appear as glinting shards of glass. What could it mean to assimilate the pieces?
Here are some examples that double down on the significance and beauty of data that is usable and brought together to paint a picture.
It’s important to note here that it’s not just integration and assimilation where Data Products play a part, but it also checks off a lot of boxes which makes the data directly valuable for the business by, say, making it accessible to a marketing team’s native tools, or qualifying the conditions set against important data features such as contact validation, or ensuring right compliance to make the data shareable for personalisation.
Let’s see a few examples of how this plays out for some specific use cases:
Ever wish you had a clearer way to prioritize leads? A data product built for lead scoring automatically ranks your prospects based on how likely they are to convert. It pulls data from various sources—like whether they’ve engaged with your emails, visited your website, or downloaded content—and assigns a score to each lead.
High scorers get more attention from your sales team, while lower scorers might need further nurturing. This data product essentially helps you focus on the most promising opportunities, ensuring that your sales team isn’t wasting time on cold leads.
One of the trickiest parts of marketing is knowing which efforts led to a conversion. Did they click an ad? Or maybe they signed up after seeing a blog post? A data product built for attribution modelling gives credit where it’s due by collecting data from all these interactions and creating a model showing the path to conversion.
It pulls in data from multiple touchpoints and creates a model that shows you the full customer journey. This way, you can invest your resources in the right places—whether that’s your email marketing or your social media ads.
Everyone loves feeling special, right? Well, a data product focused on personalisation can help you deliver that VIP experience to your customers. It takes in data like user preferences, browsing history, and purchase behaviour to serve up personalised recommendations or offers.
For example, if someone frequently checks out your social media for tips on a specific product category, a personalized email campaign can send them content that directly speaks to their interests. This makes them feel like the brand gets them, increasing the likelihood of conversion. Essentially, the data product ensures your marketing isn’t one-size-fits-all but rather custom-tailored to each person’s journey.
Well no. There is some work involved. We have to put in some fuel and strategy to get good results. But not to worry, it’s a bargain compared to traditional ops and maintenance.
Data goes through multiple layers of people, processes, and transformations before reaching the actual end-user. Every layer of people and processes comes with its own cultural bias.
We can never take the risk of ignoring this bias. Ignoring it adds inefficiencies and frustrations in every layer, making the seemingly perfect concept a painstaking undertaking that suddenly adds unbelievable friction to the data-to-consumption flow.
Products dispersed across layers take into account the concerns of users located in different layers. This is your data product stack for a marketing domain.
In the above stack, the products are coming up on demand, and the use case or purpose is at the forefront at all times. Let’s break this down a little.
Again, the aggregate products depend on how the team operates and decides to manage the constant stream of queries. How and what do marketing analysts prioritise to quench user demands?
Complex source-to-consumption transform code gets broken down into more manageable units depending on the team’s approach to code and caching. Aggregates come into the picture over time where the team realises that a conglomerate is better able to serve a broad band of queries instead of direct iteration with source products.
This becomes a new product in its own right with a separate set of objectives, resources, SLAs, and greater context given its closeness to downstream consumers.
~ Travis Thompson, Chief Architect of DataOS
These aggregates are now directly powering the data products closer to the business (Performance 360 or Lead Scoring) and enabling it more effectively than source products.
Over time, all the necessary source and aggregate products are mapped out based on consumers' usage patterns and data developers' operational comforts until you have a solid network of reliable data going up and down product funnels.
Every time data passes through a Data Product Node, the data is directly useful to the business purpose that’s next in line. This is because, by nature, the Data Product is designed to be:
Serve only the pre-defined “requirements” from the user’s end. For example, a Consumer Data Product (CDP) is built to project ROI mapping with high transparency and only integrates and works with selective data.
Checks off all pre-defined quality conditions and makes space to add new requirements.
Discoverable, understandable, and easily addressable data due to high-quality semantics added by the Data Product. Trustworthy and compliant with domain or use-case-specific policies.
Easily integrable and accessible by native tool stacks across different marketing teams.
To learn more about the details and understand how Data Products are so effective, access one of our most popular guides on the subject.
Where Exactly Data Becomes Product: Illustrated Guide to Data Products in Action
Or you could also access the more detailed playbook where we talk implementation
Data Product Platforms estimate that building individual data products could take up to 4-6 weeks (source: datadeveloperplatform.org). That’s definitely a bargain compared to years behind complex data migration and management strategies.
Each data product is “valuable on its own”, meaning it serves the case it was built for. For example, a source-aligned data product (SDP) built for broadcasting data to multiple downstream teams would do its job by providing quality-approved and governed data on demand instead of serving messy raw data directly from source.
Therefore, it’s not that you need to build a whole data product stack to observe significant value. More importantly, building a stack is a gradual process and completely requirements-dependent, as we saw above.
Triggering a collection of data products on demand is, therefore a matter of strategy as managed by Data Product Managers and the CDO.
Here’s a full picture of what a data product stack might end up looking like anywhere between 4 weeks to 3 months, depending on the strategy set forth and choice of data product platform (validate data product platforms using the community-driven standard: datadeveloperplatform.org).
At the base of the stack are SDPs, representing source-specific data from various marketing tools. These include platforms like HubSpot, Google Analytics, LinkedIn, Salesforce, Facebook Analytics, and more.
Source data is often complex, with scattered, unstructured data needing to be processed before offering value. It can get messy and disorganised when collected directly from these sources.
Sitting above SDPs are ADPs, which represent aggregated and processed data products. These integrate multiple SDPs to create holistic views of key business entities. ADPs combine, cleanse, and standardise raw data, transforming it into higher-level summaries or insights. ADPs often answer mid-tier analytical needs, such as multi-touch attribution models or customer engagement trends.
Examples:
At the top layer are Consumer-Aligned Data Products, which serve as end-user-facing data products. These are created to provide decision-makers with actionable insights by aligning with specific use cases. They deliver ready-to-use insights directly for business operations or decision-making. They take advantage of the quality-approved, governed, and aggregated data in ADPs.
These trees represent the hierarchical organisation of metrics and KPIs that evolve from niche, campaign-specific measures to broader enterprise-wide performance indicators. Each metric tree aligns with and takes advantage of different layers of the data stack, ensuring that metrics are aligned across all levels—from raw data efforts to high-level insights.
These metric trees are uber-powerful, enabling marketing teams to run extremely thorough RCA and understand what initiatives or efforts to fuel or archive. Imagine if you could connect the dots between metrics from your Content Management System, CRM, Advertising Technology, Email Campaigns and more. This gives you exponential causal awareness.
Learn more about Metrics-First Data Product Strategies here:
The Data Product Strategy - Becoming Metrics-First | Issue: #21
On the right side, we store Global Semantics to record context across the entire data ecosystem. This ensures consistency and coherence across all data products, facilitating interoperability and shared understanding. This global semantic layer (vertical, not horizontal) provides a common language for all the data products, making data across sources, aggregates, and consumer layers discoverable and interoperable.
Thanks for reading Modern Data 101! Subscribe for free to receive new posts and support our work.
From the MD101 Team 🧡
🎙️ We are stoked to introduce a brand new series 𝐀𝐧𝐚𝐥𝐲𝐭𝐢𝐜𝐬 𝐇𝐞𝐫𝐨𝐞𝐬—the ultimate podcast for analytics leaders, engineers, and analysts!
🛠 Analytics has always been, and always will be, at the heart of the data-driven world. And hence we wanted to bring the best of analytics engineering space for our community members. So join us as we dive deep with the industry's top experts to uncover insider tips, best practices, and game-changing hacks that drive real results.
📽 Each episode will feature thought leaders and seasoned data experts sharing their journey, lessons learned, and actionable insights to help you level up your analytics game. Check out the teaser to the very first episode!
If you have any queries about the piece, feel free to connect with any of the authors (details in Author Connect below). Or feel free to connect with the MD101 team directly at community@moderndata101.com 🧡
Connect with me on LinkedIn 🙌🏻
Connect with me on LinkedIn