New Release
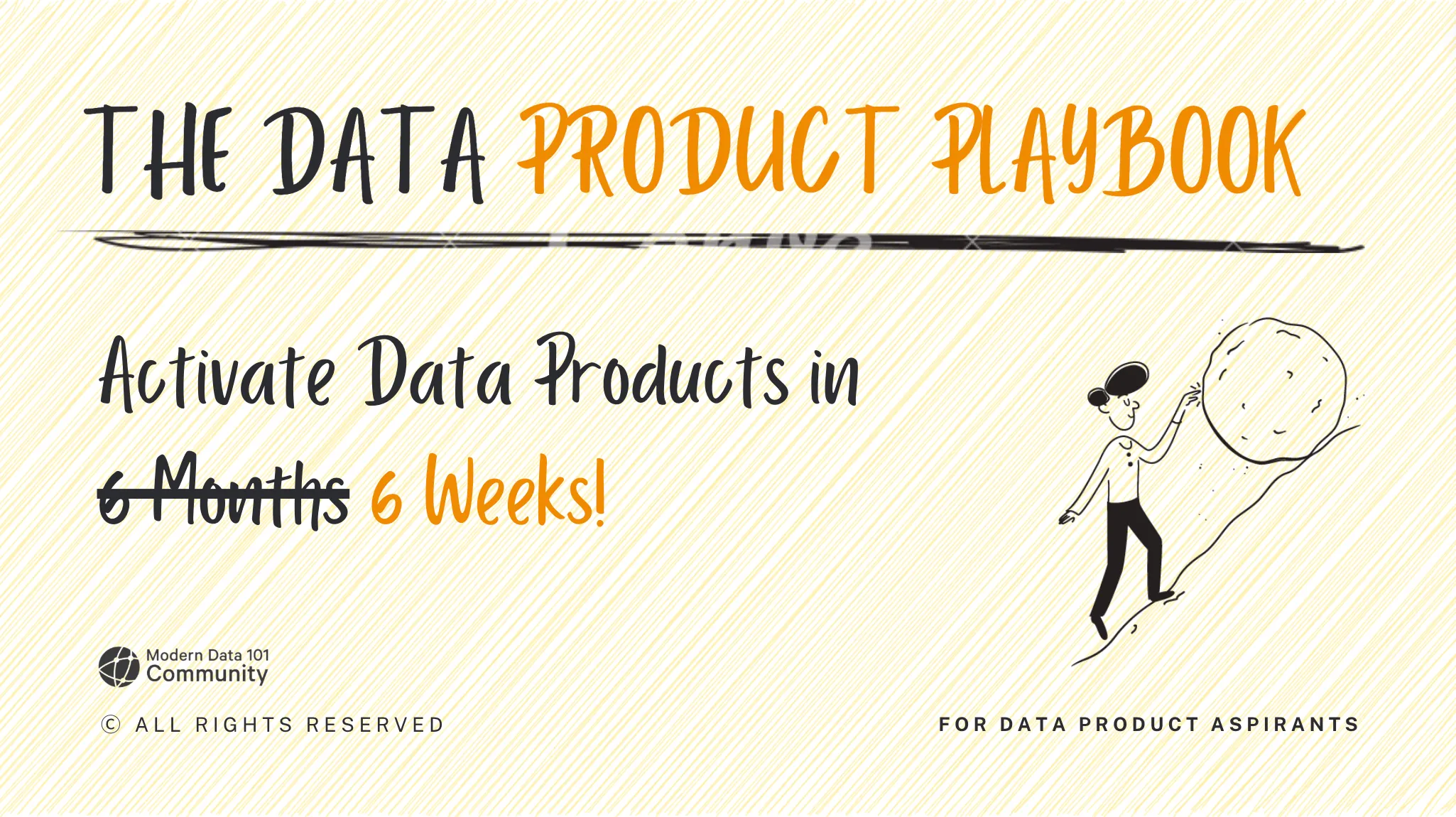
Learn More
Your submission has been received!
Thank you for submitting!
Thank you for submitting!
Download your PDF
Oops! Something went wrong while submitting the form.
Table of Contents
Get weekly insights on modern data delivered to your inbox, straight from our hand-picked curations!
the following is a revised edition.
This piece is a community contribution from Ryan Duffy, a Data Strategy Leader with over a decade of experience! He has been part of the inner workings of what it takes to effectively deliver enterprise data, including establishing the Chief Data Offices for some of the largest financial institutions – some of which are probably represented in your wallet right now. We’re thrilled to feature Ryan’s unique insights as a Strategy Leader on Modern Data 101!
We actively collaborate with data experts to bring the best resources to a 9000+ strong community of data practitioners. If you have something to say on Modern Data practices & innovations, feel free to reach out! Note: All submissions are vetted for quality & relevance. We keep it information-first and do not support any promotions, paid or otherwise!
Feature Your Ideas!
The subject of data products continues to gain traction across the industry, with some sectors leading the way while some are stepping back to learn from other’s missteps. "Data Product" has become a ubiquitous term, yet the definition varies significantly across the industry.
While numerous perspectives exist on what constitutes a data product, this article presents a practical framework for viewing data products across a spectrum from foundational to analytical methods.
In my experience as a consultant, conversations quickly get into the weeds, so I've aimed to frame the concepts of data products and the ways they can be measured to drive value into a simple construct that can then be applied and expanded further.
It is important to remember that the goal of a data product organization is not only to build data products but also to measure, manage, and understand...
…how their Data Product Portfolio is being used to achieve top-line business goals.
At the base of the data product spectrum are foundational data products - the bedrock of enterprise data architecture. These domain-oriented products serve as the authoritative source of master and reference data.
Think of a financial institution's customer master data product containing the golden source of customer information or a product master maintaining definitive product specifications and hierarchies.
These foundational products are made available through data marketplaces (i.e., internal platforms that enable controlled discovery, access, and distribution of data products across the organization), enabling broad enterprise consumption while maintaining data governance standards (i.e., the policy and procedures used as organizational rules and processes for data management).
The foundational products are often the first to be created as they are more discrete, and many other products and services use them as clean, trusted data sources.
Note: While I am using the term “Foundational Data Product” this has also been referenced using similar terms within the industry such as “Source Data Products” or “Entity Data Products” as cited by other community contributors (e.g., referenced in articles [1], [2], [3])
Moving along the spectrum, the next bucket of data products can be described as integrated and combine data across domains to serve specific business needs.
For instance, a "Customer 360" data product might merge customer master data with transaction history, product holdings, and interaction data.
These products are purpose-built but maintain flexibility for various use cases. They serve as building blocks for more sophisticated analytical solutions while providing immediate value through their integrated view of cross-domain datasets.
Note: Similar to multiple terms referencing foundational data products, “Integrated Data Products” have also been referenced using similar terms within the industry such as “Aggregated Data Products”, “Derived Data Products” or “Business Data Products” as cited by other community contributors (e.g., referenced as Aggregated here.)
At the far end of the spectrum are analytical data products. These are less ‘data as a product’ type dataset products, but rather purely consumable assets that are designed to drive action with specific insights, answer questions, or solve use cases using the other data products. These take various forms:
Note: As with other data products, other Modern Data 101 contributors have offered insights on this topic as well. Learn more here!
With this understanding of the data product spectrum, organizations need to evaluate and improve their data products systematically in a way that maintains high value and relevance.
Having data products for the sake of having data products is a losing strategy. The following proposed measurement framework provides the structure needed to assess and enhance data products across all levels of the spectrum.
“Do we have a consistent way to measure and identify tangible business value?”
Inventorying data products, classifying them by domain or business, and knowing counts and distribution is a great start to begin managing the portfolio, but measuring data products isn't just about tracking metrics—it's about driving and understanding value. While organizations continue to gain traction in building data products, particularly on the analytical side of the spectrum, many often struggle to measure their effectiveness systematically.
In nearly every organization I've been part of or consulted for, countless dashboards and curated data sets exist in varying stages of maturity, use, and age. The exponential growth is a result of treating data solutions as 'projects' rather than 'products' that continually evolve to meet new demands and maintain relevancy.
📝 Related Reads
Building your Sausage Machine for Data Products 🌭: Less Tech, More Strategy | Issue #37
This challenge stems from the complexity of tracking not just technical performance but also business value and user satisfaction across different types of products along the spectrum. Consistent measures must be in place to monitor and evolve your data product portfolio.
A comprehensive measurement framework serves multiple purposes.
To create an effective measurement system, organizations need to think holistically about their data products. This means moving beyond simple usage statistics - which, again, is a great start and better than nothing - to understand the full picture of product health, adoption, and performance.
By integrating these measurements into a comprehensive scorecard, organizations can assess their data product portfolio at multiple levels moving from individual products to domain-specific collections to enterprise-wide analytics.
A scorecard approach enables teams to identify under-performing products quickly, celebrate successes, and make data-driven decisions about product investments.
For instance, a data domain owner might discover that while their foundational data products have high-quality scores, adoption rates are low due to poor documentation or accessibility issues. Similarly, an enterprise view might reveal overlapping products across domains, presenting opportunities for consolidation and cost savings.
📝 Related Reads
Metrics-Focused Data Strategy with Model-First Data Products | Issue #48
The Go-To Metrics for Data Products: Start with these Few | Issue #12
How does a Data Product Strategy Impact the Day-to-Days of Your CMO, CDO, or CFO | Issue #34
"Is the product trusted and described correctly?"
The cornerstone of any successful data product or program lies in its trustworthiness and clarity of purpose. This measurement bucket focuses on the fundamental quality and documentation aspects that determine whether a data product can be reliably used as a source of truth.
By monitoring health metrics, organizations can ensure their data products maintain the highest standards of data integrity and usability.
Quantitative Measures
Qualitative Measures