New Release
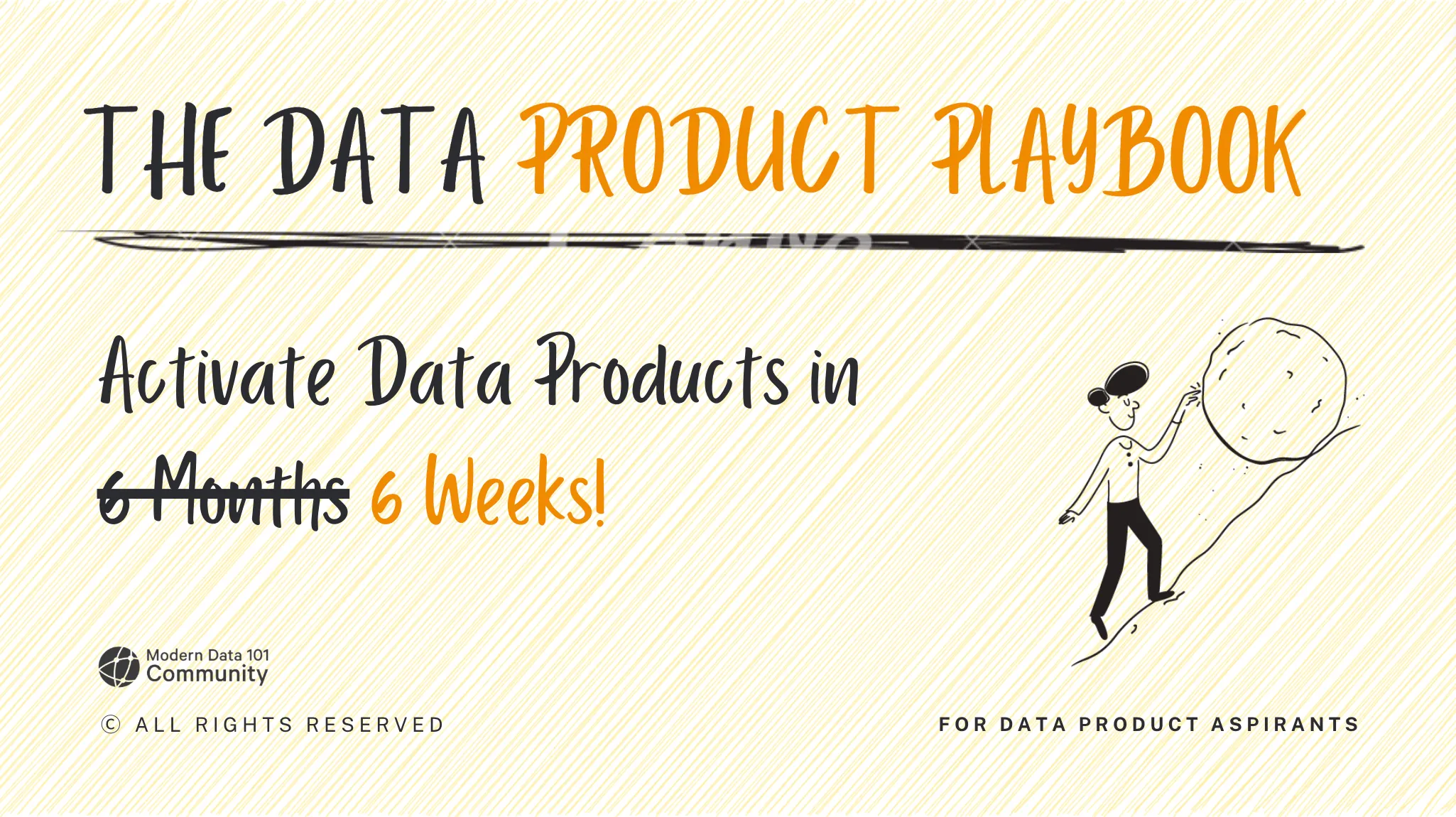
Learn More
Your submission has been received!
Thank you for submitting!
Thank you for submitting!
Download your PDF
Oops! Something went wrong while submitting the form.
Table of Contents
Get weekly insights on modern data delivered to your inbox, straight from our hand-picked curations!
the following is a revised edition.
The concept of "Data as a Product" has become the standard (or at least the buzzword) in the data management industry. Its fundamental characteristics are encapsulated in the acronym DATSIS: Discoverable, Addressable, Trustworthy, Secure, Interoperable, and Self-Describing. These elements are becoming the goal and the north star for various data architectures.
Today, I would like to pause and talk about, even at the risk of playing with nuances, the concept of "Trustworthy" because, in its mainstream conception, it might be limiting new solutions, bringing them back to the warehousing era (and those who know me know how much of a supporter I am of warehousing).
To address this topic, it is important to introduce two key supporting concepts:
A data product is essentially composed of data (data assets) and metadata (self-descriptors and data usage & policies) that describe its characteristics and access methods.
📝 Related Read:
The 3 Data Product Components 💠
In the physical world, not all products are created for the same uses. There are generalist products and technological products with specific characteristics.
📝 Related Read:
Model-First Data Products and How They Enable Different Business Purposes
In discussions, I am noticing that the concept of "Trustworthy" is often incorrectly considered synonymous with "certified" or "best of breed". Domains populate few, very few data products, and these are often equivalent to a Master Data Management (MDM) or a certified Data Mart, following long (and costly) processes of data engineering and manipulation.
Instead, we should adopt a concept of "good enough" for the level of trust. This statement, which may seem quite "bold," actually makes much more sense when related to the world of physical products (not digital or informational).
To support this discussion, let's take a common example that we all know: footwear (an example useful in several other aspects as well). We can consider dividing these products into three main categories that can be mapped on the medallion architecture layer:
Not applying this reasoning to the world of information management is therefore limiting because it restricts us to using only products that serve a specific and narrow purpose and limits us in accessing a much broader wealth of information.
This self-limitation becomes even more evident when we consider that in data platforms, the gold layer is often an infinitesimal fraction of the total information assets (both in terms of the number of objects and volume).
The question then becomes: why should we limit interoperability to a fraction of the possibilities while simultaneously increasing operational costs? The answer is that raw data does not allow for complete ownership and accountability of the solution.
📝 Editor’s Note
Learn more about designing Data Products for different consumption patterns/layers:
How to Build Data Products - Design: Part 1/4 | Issue #23
Beyond the need for a cultural shift to overcome the obstacle of sharing only "perfect and validated" data, there is also an issue of process and real accountability. Business Data Owners (BDOs) and Data Product Owners (DPOs) are typically held accountable and responsible for their data in its most stringent form.
However, this creates a boomerang effect where BDOs and DPOs tend to publish only well-maintained data to avoid subsequent extra effort in managing defects and maintenance.
To manage this issue, we need to consider the previously introduced triad (asset + self-descriptor + data usage policies). It is precisely in the union of these three factors, and specifically in the policies that we can work to enable a usage mode that integrates with the risk-free approach.
A pragmatic approach is to blur the ownership of the data into an advisory and/or guidance role, even for silver data, and then effectively dissolve it, at least for the business component, for access to bronze data.
By doing so, we could ensure access to the entirety of the informational assets without burdening BDOs with an ungrateful and possibly unnecessary task (when certified data is required, one should go for a higher-quality Data Product).
The approach described above supports a series of situations (calling them use cases might be an overstatement) that I encounter daily:
To enable this scenario, it is necessary to:
In conclusion, embracing a more nuanced and flexible approach to data trustworthiness is crucial for unlocking the full potential of our data assets. By recognising that not all data needs to meet the highest standards of certification and allowing for varying levels of trust, we can significantly expand the usability and interoperability of our data products.
This shift not only reduces operational costs but also encourages innovation and efficiency across data-driven projects. As we move forward, redefining the roles and responsibilities of Business Data Owners and implementing robust data policy management systems will be key to fostering a more inclusive and effective data ecosystem. By doing so, we can ensure that our data platforms are not just reservoirs of information but dynamic environments that drive growth and value.
🧺 More from the Author
EXPERT'S DESK: Bringing Home Your Very First Data Product