New Release
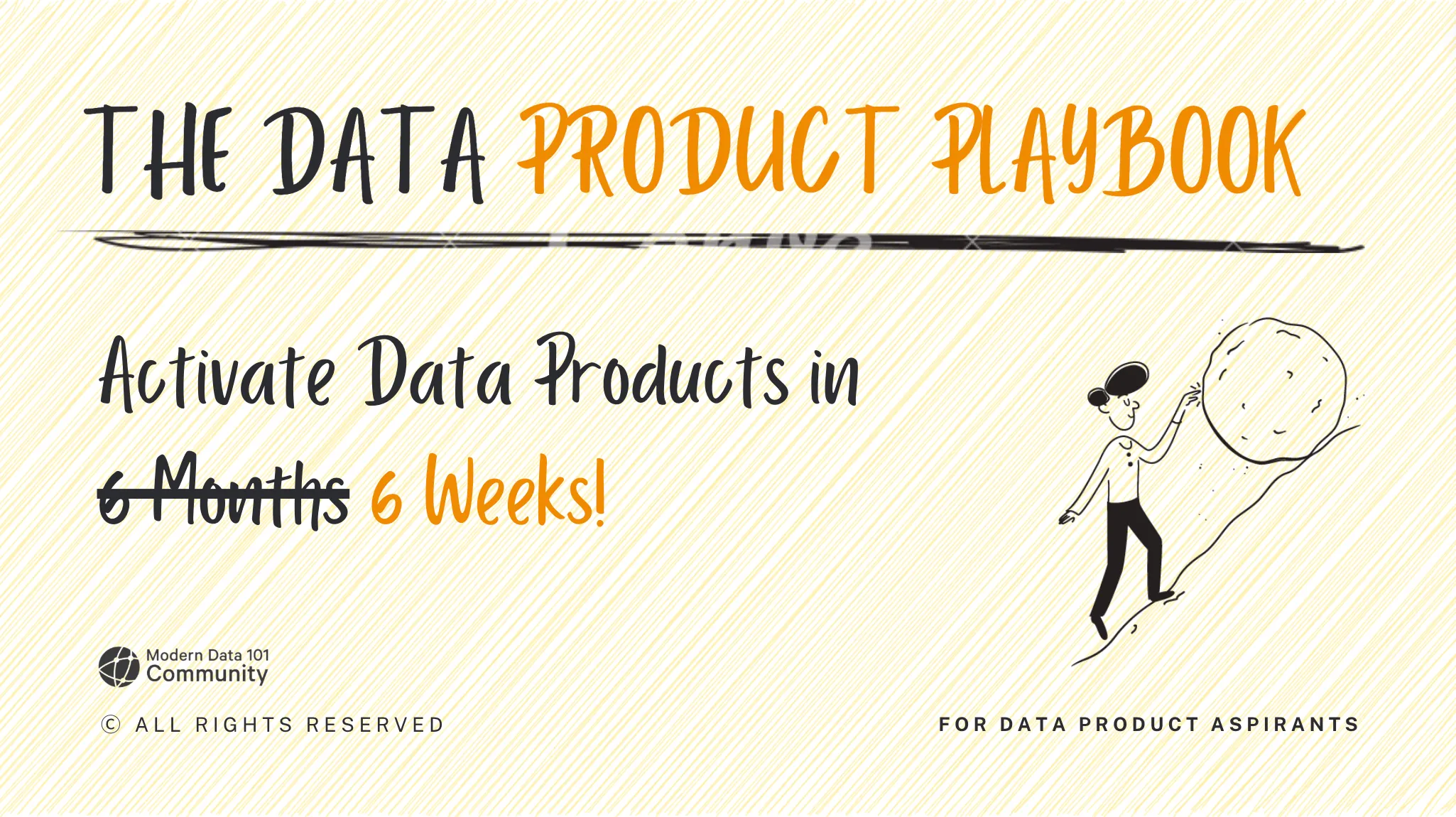
Learn More
Your submission has been received!
Thank you for submitting!
Thank you for submitting!
Download your PDF
Oops! Something went wrong while submitting the form.
Table of Contents
Get weekly insights on modern data delivered to your inbox, straight from our hand-picked curations!
the following is a revised edition.
Before diving in, a very Happy New Year 🍻🎉 to You from Modern Data 101! As always, we’re looking forward to concocting more amazing ideas with you this year and beyond! Entering 2025 like ➡️
This piece is a community contribution from Francesco, an expert craftsman of efficient Data Architectures using various patterns. He embraces new patterns, such as Data Products, Data Mesh, and Fabric, to capitalise on data value more effectively. We highly appreciate his contribution and readiness to share his knowledge with MD101.
We actively collaborate with data experts to bring the best resources to a 9000+ strong community of data practitioners. If you have something to say on Modern Data practices & innovations, feel free to reach out!
🫴🏻 Share your ideas and work: community@moderndata101.com
*Note: Opinions expressed in contributions are not our own and are only curated by us for broader access and discussion. All submissions are vetted for quality & relevance. We keep it information-first and do not support any promotions, paid or otherwise!
TOC
Differences b/w Federated and Centralised Modeling
Drivers for the Business Case: Qualitative & Quantitative
Analysis of Economic Advantages
Considerations and Key Takeaways
The adoption of data mesh paradigms and federated data management is currently at the peak of the hype cycle. However, it is essential to recognize that these approaches not only represent an evolution in operational models but also come with significant operational costs. These costs can lead to unsustainable efforts in the initial stages and, eventually, to financial strain if not properly managed.
The goal of this article is to analyze the key drivers to consider when quantifying the most suitable operational model for a given context. The aim is to prioritize practicality and informed decision-making, even at the expense of strict methodological purity.
The concept of a federated data modeling team encourages a collaborative approach to managing and utilizing data across various organizational sources. While this model mitigates some of the challenges traditionally encountered by centralized teams, it also introduces new responsibilities and tasks, as outlined in the table below.
It is worth highlighting what I see as the "elephant in the room": security and governance activities, which, based on my experience, are often not actively embraced by data modeling teams. Within a Domain-Driven Design (DDD) approach, however, these responsibilities can no longer be avoided or delegated elsewhere.
As with any business case, we can classify the advantages into quantitative (both economic and non-economic) and qualitative categories.
Quantitative Advantages - Economic:
Quantitative Advantages - Non-Economic:
Qualitative Advantages
In this exercise, we will focus solely on the quantification of economic advantages, with the addition of the “% Reuse” metric. The rationale is that reuse directly enables the calculation of avoided costs associated with duplicate data assets, which is critical for the success of the business case.
On the other hand, “Time to Market”, while important, is difficult to quantify beyond localized contexts and does not add significant value to the storytelling in this scenario. For this reason, it will not be included in this analysis, which will instead be conducted using realistic values specific to Northern Italy and cloud-native solutions with a single cloud provider.
Geographic changes or architectural shifts could, of course, alter the calculation coefficients, as we will see later. However, to maintain confidentiality, these values will be anonymized and represented using Monopoly money (or “duck-dollars” 🦆)
In this section, we’ll calculate a few scenarios to assess effort, implementation, and operational costs. The goal is to highlight key takeaways ("so what") and provide insights into the practicality using the following inputs:
These initial considerations must be complemented by an assessment of the required effort, as well as the implementation and operational costs. While the implementation and operational values will naturally be expressed in duck-dollars 🦆 (and thus remain implicit), I believe the allocation framework is sufficiently generic to be broadly applicable.
The first scenario considers the following drivers based on my observations with clients of medium maturity:
These drivers plugged into the incredibly complex simulation engine (called MS Excel :) ), yield a detailed and cumulative valuation, with a breaking point occurring in the second and fourth years, as represented below in proportion to the platform's total cost (TOTEX).
Even this initial result, although quite conservative, could be significant on its own. However, it is important to emphasize that by analyzing the cost breakdown, we can observe how the investment decreases proportionally over time due to the diminishing incremental costs of the platform.
This brings us to the first key takeaway: federated modeling is not a sprint but rather a marathon.
It is also important to emphasize that the numbers provided above represent a baseline that is easily achievable without excessive commitment. However, the model can be altered by leveraging different decision-making levers:
Somewhat counterintuitively, these actions are more likely to impact the scale of the return rather than the breaking event, which remains largely unaffected as it is tied to the availability of data provided by users.
Before moving to the conclusions, however, it is important to make an underlying assumption explicit: the availability within the team (in-house or otherwise) of competent and, above all, dedicated data modelers. While the results are certainly promising, they presuppose the establishment of a potentially significant workforce. If this effort is not adequately sponsored, it may fail to attract the necessary talent.
To provide additional clarity, the following is a diagram of the distribution.
While this is a partially stylistic exercise, it remains significant as it models a series of critical phenomena:
Success requires not only technological elements (catalog, marketplace) but, most importantly, strong sponsorship, ideally formalized in a process. This sponsorship must see modelers as active participants rather than passive "blessing" figures for initiatives.
For the sake of brevity, I had to condense certain points. Let me know if there’s a need to elaborate or delve deeper, perhaps in a follow-up article.
Thanks for reading Modern Data 101! Subscribe for free to receive new posts and support our work.
If you have any queries about the piece, feel free to connect with the author(s). Or feel free to connect with the MD101 team directly at community@moderndata101.com 🧡
Find me on LinkedIn 🤝🏻